Importing packages – Pipelines using TensorFlow Extended-2
Transform:
We will create file_transform.py which will contain information about the data labels and feature engineering steps:
• Run the following mentioned code in new cells to declare variable containing file name:
TRANSFORM_MODULE_PATH = ‘file_transform.py’
• Run the following mentioned codes in a new cell to create file_transform.py file. Preprocessing (preprocessing_fn) function is where the actual alteration of the dataset occurs. It receives and returns a tensor dictionary, where tensor refers to a Tensor or SparseTensor. In our example, we are not applying any transformations, code is just mapping to the output dictionary. (%%writefile command will create the .py file with the following code in it.):
%%writefile {TRANSFORM_MODULE_PATH}
import tensorflow as tf
import tensorflow_transform as tft
NAMES = [‘AF3’,’F7’,’F3’,’FC5’,’T7’,’P7’,’O1’,’O2’,’P8’,’T8’,’FC6’,’F4’,’F8’,’AF4’]
LABEL = ‘eyeDetection’
def preprocessing_fn(raw_inputs):
processed_data = dict()
for items in NAMES:
processed_data[items]=raw_inputs[items]
processed_data[LABEL] = raw_inputs[LABEL]
return processed_data
- Files needs to copy into GCS bucket, run the following line of code to copy it:
!gsutil cp file_transform.py {MODULE_FOLDER}/ - Run the following mentioned lines of codes in a new cell to configure transform component. Transform component is taking inputs from the example_gen and schema_gen:
transform_data = tfx.components.Transform(
examples=example_gen_csv.outputs[‘examples’],schema=gen_schema.outputs[‘schema’],
module_file=os.path.join(MODULE_FOLDER, TRANSFORM_MODULE_PATH))
context_in.run(transform_data, enable_cache=False)
Output of the transform component is as shown in Figure 8.10. Transform graph is one of the artifacts generated by the transform component and it will be used for the trainer module.
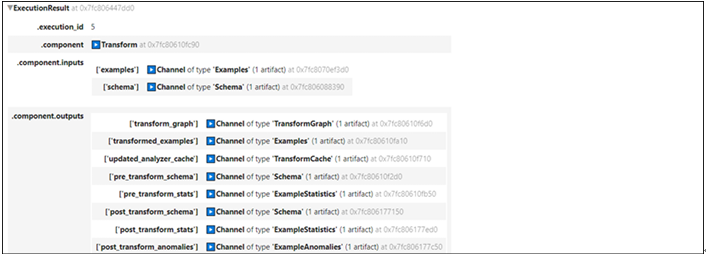
Figure 8.10: Output of transform component
Run the following mentioned codes to check few records of the transformed data (This code will not be needed during the pipeline construction):
train_sam = os.path.join(transform_data.outputs[‘transformed_examples’].get()[0].uri,’Split-train’)
filenames_tfr = [os.path.join(train_sam, name) for name in os.listdir(train_sam)]
dataset = tf.data.TFRecordDataset(filenames_tfr, compression_type=’GZIP’)
for record in dataset.take(1):
sample = tf.train.Example()
sample.ParseFromString(record.numpy())
print(sample)
Output of 1 record will be as shown in Figure 8.11:
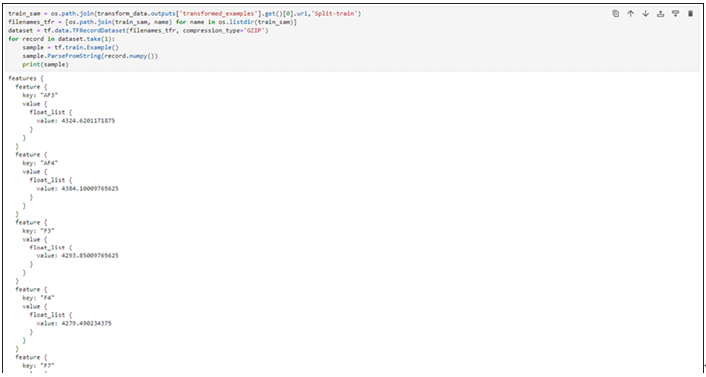
Figure 8.11: Transformed data example
Trainer:
The Trainer component is in charge of preparing the input data and training the model. It requires the ExampleGen examples, the transform, and the training code. TensorFlow Estimators, Keras models, or custom training loops can be used in the training code. When compared to other components, trainer requires more modifications in the code:
• Run the following mentioned code in the new cell, it will generate trainer.py file (Complete code is available in the repository). Trainer.py (training code) file will be the input for the trainer module. Trainer component generates two artifacts model (trained model itself) and modelrun which can be used for storing logs, this can be seen in Figure 8.12. The Trainer.py file contains four functions; high level description of those functions is:
o run_fn will be entry point to execute the training process
o _input_fn generates features and labels for training
o _get_serve_tf_examples_fn returns a function that parses a serialized tf.example
o _make_keras_model creates and returns the model for classification
%%writefile trainer.py
from typing import List
from absl import logging
import tensorflow as tf
import tensorflow_transform as tft
from tensorflow import keras
from tensorflow_transform.tf_metadata import schema_utils
from tfx import v1 as tfx
from tfx_bsl.public import tfxio
from tensorflow_metadata.proto.v0 import schema_pb2
COL_NAMES=[‘AF3’,’F7’,’F3’,’FC5’,’T7’,’P7’,’O1’,’O2’,’P8’,’T8’,’FC6’,’F4’,’F8’,’AF4’]
LABEL=”eyeDetection”
BATCH_SIZE_TRAIN = 40
BATCH_SIZE_EVAL = 20
def _input_fn(files,accessor,transform_output,size) -> tf.data.Dataset:
Creates datasets and apply transformations on them and return.
Refer the repository for code block of the function
return dataset.map(apply_transform_fn).repeat()
def _get_serve_tf_examples_fn(model, transform_output):
To parse the serialized examples and return.
Refer the repository for code block of the function
return serve_tf_examples_fn
def _make_keras_model() -> tf.keras.Model:
Create model with layers, loss functions to be used and return.
Refer the repository for code block of the function
return model_classification
def run_fn(fn_args: tfx.components.FnArgs):
tf_transform = tft.TFTransformOutput(fn_args.transform_output)
train_samples = _input_fn(_args.train_files, _args.data_accessor, tf_transform, =BATCH_SIZE_TRAIN)
eval_samples = _input_fn(_args.eval_files, _args.data_accessor, tf_transform, =BATCH_SIZE_EVAL)
model_classification = _make_keras_model()
model_classification.fit(
train_samples,
steps_per_epoch=fn_args.train_steps,
validation_data=eval_samples,
validation_steps=fn_args.eval_steps)
sign = {
“serving_default”: _get_serve_tf_examples_fn(model_classification, tf_transform),
}
model_classification.save(fn_args.serving_model_dir, save_format=’tf’,signatures=sign)
- Run the following code to copy the trainer.py to GCS storage:
!gsutil cp trainer.py {MODULE_FOLDER}/ - Run the following code to initiate the trainer component. trainer_file=”trainer.py”:
trainer_file_path=os.path.join(MODULE_FOLDER, trainer_file)
model_trainer = tfx.components.Trainer(
examples=example_gen_csv.outputs[“examples”],
transform_graph=transform_data.outputs[“transform_graph”],
train_args=tfx.proto.TrainArgs(num_steps=200),
eval_args=tfx.proto.EvalArgs(num_steps=10),
module_file=trainer_file_path,
)
context_in.run(model_trainer, enable_cache=False)
The output of the trainer component is as shown in the following figure:
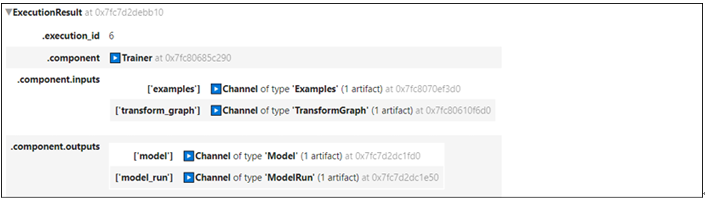
Figure 8.12: Output of the trainer component