Fetching feature values – Vertex AI Feature Store
Step 12: Fetching feature values
Feature values can be extracted from the feature store with the help of an online service client which has been created in Step 7 (Creation of feature store). Run the following lines of code to fetch data from the feature for a specific employee ID:
resp_data = client_data.streaming_read_feature_values(
featurestore_online_service.StreamingReadFeatureValuesRequest(
entity_type=client_admin.entity_type_path(
Project_id, location, featurestore_name, Entity_name
),
entity_ids=[“65438”],
feature_selector=FeatureSelector(id_matcher=IdMatcher(ids=[“employee_id”,”education”,”gender”,”no_of_trainings”,”age”])),
)
)
print(resp_data)
The output will be stored in the resp_data variable and it is an iterator. Run the following lines of code to extract and parse the data from the iterator:
names_col=[]
for resp in resp_data:
if resp.header.feature_descriptors != “”:
for head in resp.header.feature_descriptors:
names_col.append(head.id)
try:
values=[]
for items in resp.entity_view.data:
if items.value.string_value !=””:values.append(items.value.string_value)
elif items.value.int64_value !=””:values.append(items.value.int64_value)
except:pass
print(“Feature Names”,names_col)
print(“Feature Values”,values)
The output of the cell is shown in Figure 9.21:
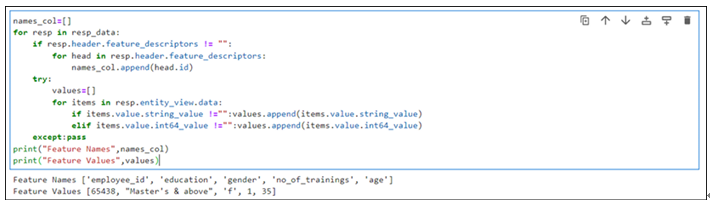
Figure 9.21: Data extracted from the feature store
Deleting resources
We have utilized cloud storage to store the data and delete the CSV file from the cloud storage manually. Feature store is a cost-incurring resource on GCP, ensure to delete them. Also, the feature store cannot be deleted from the web console or GUI, we need to delete it through programming. Run the below-mentioned lines of code to delete the feature store (both the feature stores were created using GUI and Python). Check if the landing page of the feature store after running the code to ensure it is deleted (the landing page should look like the Figure 9.4):
client_admin.delete_featurestore(
request=fs_s.DeleteFeaturestoreRequest(
name=client_admin.featurestore_path(Project_id, location, featurestore_name),
force=True,
)
).result()
featurestore_name=employee_fs_gui
client_admin.delete_featurestore(
request=fs_s.DeleteFeaturestoreRequest(
name=client_admin.featurestore_path(Project_id, location, featurestore_name),
force=True,
)
).result()
Best practices for feature store
Below listed are few of the best practices for using Feature store of Vertex AI:
- Model features to multiple entities: Some features might be used in multiple entities (like clicks per product at user level). In this kind of scenarios, it is best to create a separate entity to group shared features.
- Access control for multiple teams: Multiple teams like data scientists, ML researchers, Devops, and so on, may require access to the same feature store but with different level of permissions. Resource level IAM policies can be used to restrict the access to feature store or particular entity type.
- Ingesting historical data (backfilling): It is recommended to stop online serving while ingesting the historical data to prevent any changes to the online store.
- Cost optimization:
- Autoscaling: Instead of maintaining a high node count, autoscaling allows Vertex AI Feature Store to analyze traffic patterns and automatically modify the number of nodes up or down based on CPU consumption and also works better for cost optimization.
- Recommended to provide a startTime in the batchReadFeatureValues or exportFeatureValues request to optimize offline storage costs during batch serving and batch export.
In this chapter, we learned about the feature store of Vertex AI, and worked on the creation of the feature store, entity type, adding features, and ingesting feature values using web console and Python.
In the next chapter, we will start understanding explainable AI, and how explainable AI works on Vertex AI.
- What are the different input sources from which data can be ingested into a feature store?
- Can feature stores have multiple entity types?
- What are the scenarios in which using a feature store brings value?